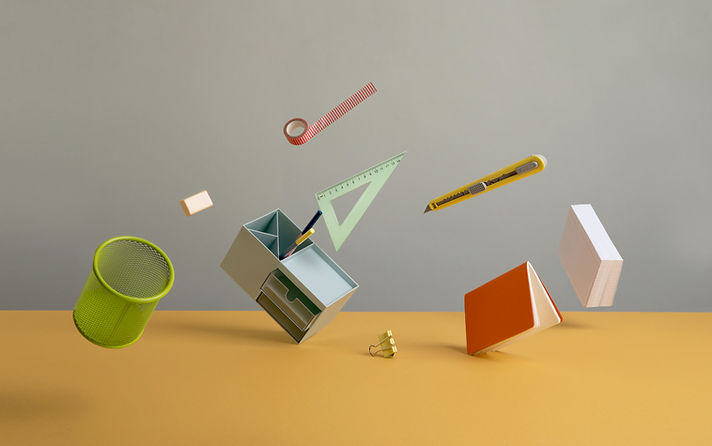
educational research
Beginning in January of 2017, Doherty began a collaboration with Denis Dumas (Ph.D, Educational Psychology) at the University of Denver. Over a series of five articles, printed across five peer-reviewed journals, the team studied the personality traits and thought processes of professional actors.
​
[Published February 2020 in Psychology of Aesthetics Creativity and the Arts]
by Denis Dumas, Peter Organisciak, and Michael Doherty
Genesis: The team distributed an Alternate Uses Task (e.g. "How many uses for a brick can you think of in two minutes?") to measure creativity in hundreds of actors, and now had to generate Originality scores for all of their responses.
​
Problem: Though human raters could perhaps generate the most accurate Originality score, that would require a lot of time and resources.
​
Solution: There are a number of text-mining models, or language datasets, that can measure Originality.
Study: The team used most popular models to determine which one was the most effective.
​
Findings: Human raters were, indeed, the most reliable in rating Originality, but the language dataset GloVE 840B was a close second, making it the most reliable system to measure Originality in artists.
The Psychology of Professional and Student Actors: Creativity, Personality, and Motivation
​
[Published October 2020 in PLoS ONE]
by Denis Dumas, Michael Doherty, and Peter Organisciak
Genesis: The team was curious about how actors' brains work, and therefore wanted to learn the psychological and neurological differences between them and other people.
​
Problem: After collecting data from professional actors, student actors, and non-actors, the data the team collected was difficult to compare in meaningful ways.
​
Solution: By using a classification model, in this case an actor-sorting artificial intelligence (AI) system, the team could determine which differences between actors and non-actors were significant enough to distinguish the two groups.
​
Study: Use said AI system to determine the key differences between actors and non-actors, and between professional actors and student actors.
​
Findings: The AI system was able to distinguish actors from non-actors with a 92% accuracy, and found that actors had higher levels of Extraversion, Openness, Assertiveness, Elaboration, and Creative Activities. It distinguished professional actors from student actors with a 96% accuracy (68% when the team didn't factor in age), and found that professional actors high higher levels of Originality, Volatility, and Literary Activities.
The Influence of Creative Expertise on the Sensitivity and Selectivity of Analogical Reasoning
[Published March 2021 in Mind Brain and Education]
by Denis Dumas, Yixiao Dong, and Michael Doherty
Genesis: The team administered an Analogy Finding Task (i.e. Complete the Analogy) to their participants, and measured both their ability to make a successful analogy (Sensitivity) and their ability to rule out non-valid analogies (Selectivity).
​
Problem: The team wanted to ensure that all participants were able to use their imaginations in the AFT, regardless of creative expertise.
​
Solution: All participants were asked to do the task, and then asked to do it while "thinking creatively."
​
Study: Determine how the "think creatively" prompt affected actors versus non-actors.
​
Findings: While the "think creatively" prompt increased actors' Sensitivity much more than non-actors, it also decreased their Selectivity much more than non-actors. The team's hypothesis is that this is due to an actor's flexibility when given a creative prompt.